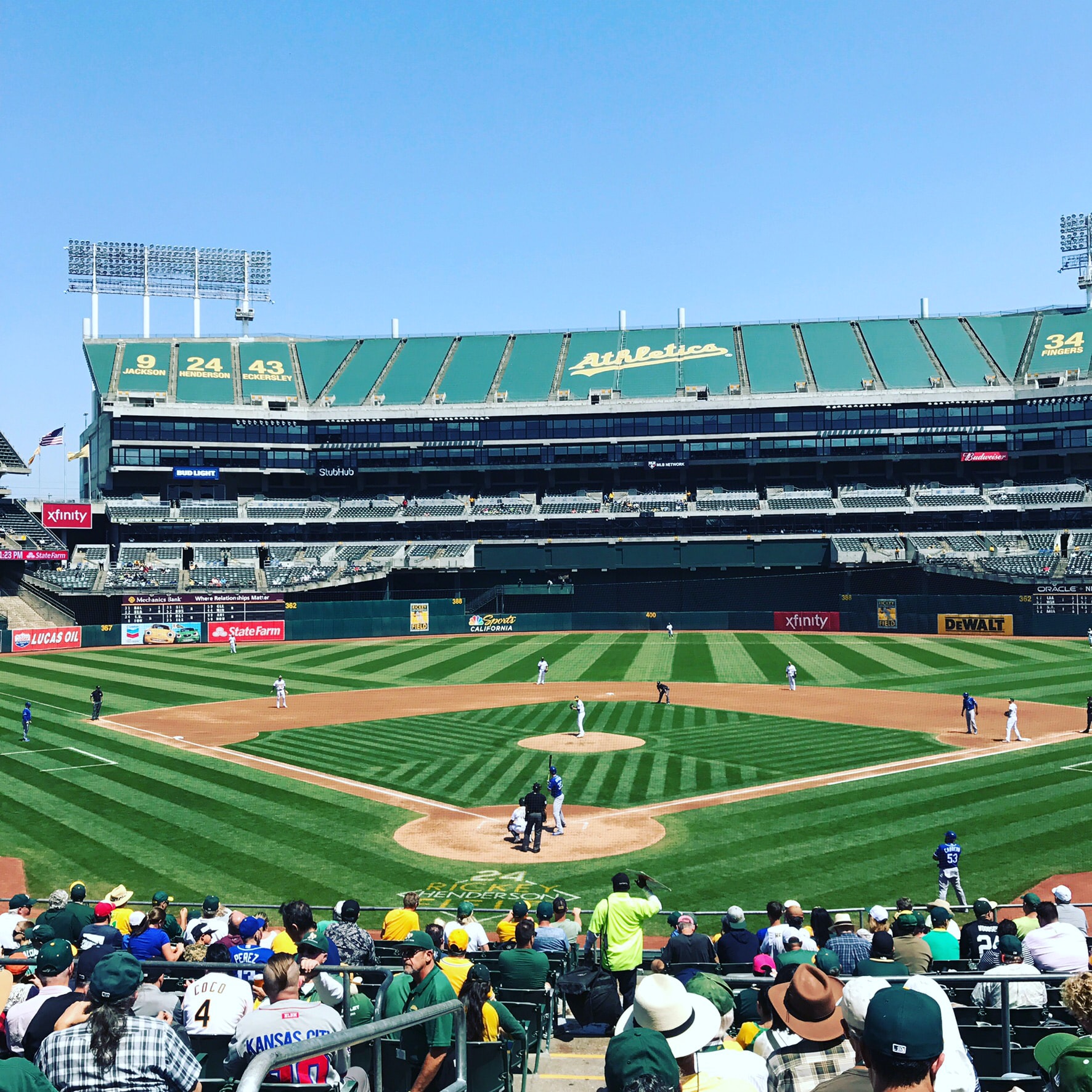
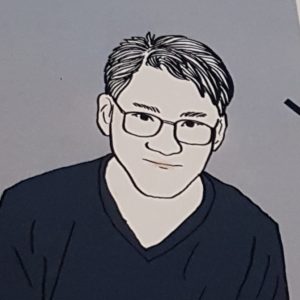
Wongsakorn Watch.
Master of Electrical Engineering (Telecommunication)
Master of Business Administration (Finance)
More than 20 years in Telecommunication business
อ่านแล้วชอบ เพื่อเป็นกำลังใจ
ช่วย กดLike กดShare ด้วยนะครับ
Analytics in Sport
Analytics กับ การกีฬา
- May 26, 2020
- , 5:18 pm
- , Analytics
โพสต์นี้เป็นการนำ Analytics มาใช้กับกีฬาเบสบอล จากภาพยนตร์เรื่อง Moneyball 2011 โดย Brad Pitt รับบทเป็นนักแสดงนำ
ผมต้องดูหนังเรื่องนี้ถึงสองรอบเพราะเป็นหนังที่ดีมากจริงๆ
ผมไม่ลงรายละเอียดของหนังแต่ จะเขียนในเนื้อหาที่เกี่ยวกับ Analytics เท่านั้นครับ
This article gives an example of how Analytics can be used to make better decisions about baseball. This article has been inspired by Moneyball 2011, Brad Pitt as Billy Beane.
It’s a great movie. To me, I don’t know much about baseball rules so it is not easy to understand all of them.
I will try not to enter the details of the movie, but only reveal in the content related to Analytics.
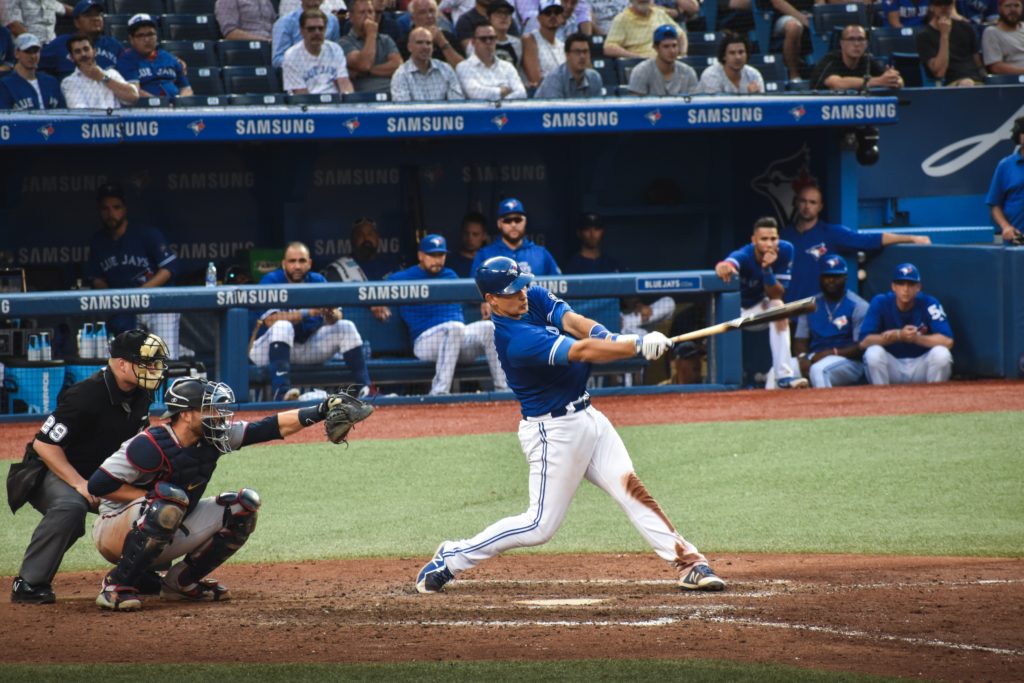
Unfair Game
คำว่า unfair game คือ ทีมที่รวยกว่าย่อมได้ผู้เล่นที่ดีกว่า และ จะชนะมากกว่า
Oakland A’s เป็นทีม Baseball ที่มีเงินน้อยในการซื้อตัวผู้เล่นเก่งๆที่มีค่าตัวแพง
Billy Beane ผู้จัดการทีม (แสดงนำโดย Brad Pitt) คิดอย่างหนักว่าจะทำอย่างไรที่จะก้าวข้ามผ่านปัญหานี้ได้
Billy Beane จึงตัดสินใจใช้ Analytics สู้กับปัญหานี้
เขาทำอย่างไร?
Oakland A’s is a baseball team that has a very limited budget to buy players, and good players have a high price. Billy Beane, as the manager, has a deep thought about how to overcome this problem.
The word unfair game is that a richer team gets better players and wins better.
But Oakland A’s has a different way of thinking, and Oakland A’s, led by manager Billy Beane, uses Analytics to fight the problem.
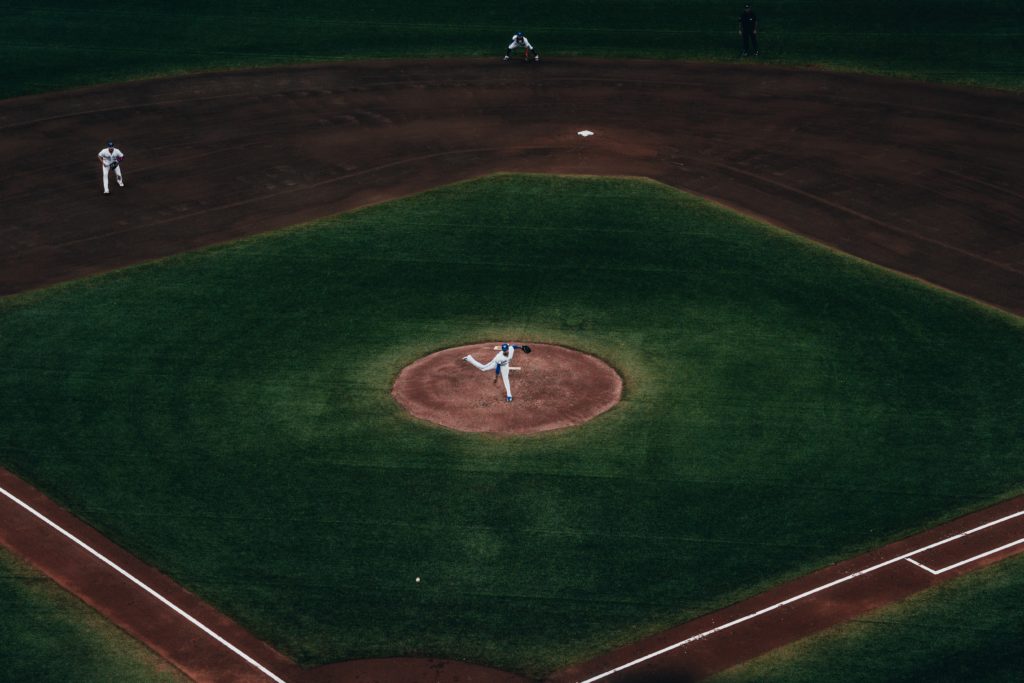
Illusion is the cause of unfair game problem.
ในอดีตการคัดเลือกนักกีฬา แมวมองมีเงื่อนไขในการเลือกผู้เล่น เช่น
เปอร์เซ็นต์การตีได้ดี, หน้าตาดีจะมีความมั่นใจในการเล่น, มีสรีระที่ดีทำให้สามารถสร้างสมดุลของร่างกายได้ดี การมีวงสวิงที่สวยงาม หรือ มีท่วงท่าในการขว้างลูกที่สวยงาม
Billy Beane รู้ดีว่าสิ่งที่แมวมองคิด ไม่สามารถเอาชนะทีมที่มีเงินเยอะได้ ซึ่ง ไม่ใช่วิธีการแก้ปัญหาเรื่อง unfair game.
Billy Beane ถามแมวมองในที่ประชุมซ้ำๆ ว่า พวกคุณรู้หรือยังว่าปัญหาคืออะไร? ดังนั้นสิ่งสำคัญในการทำ Analytics คือการมองปัญหาให้เจอ และ หาสาเหตุของปัญหาให้ได้
In the past, the selection of athletes, Scouts have conditions for choosing, such as a good percentage of hits, good looks, confidence in playing, good physiology, which can create a good balance of the body. Having a beautiful swing.
Billy Beane knows that the scout opinions are not true (why Billy Beane thinks like that? find the answer in the movie) and not a solution to fix the unfair game.
For Analytics, it is very important to find the problem and the causes of the problem.
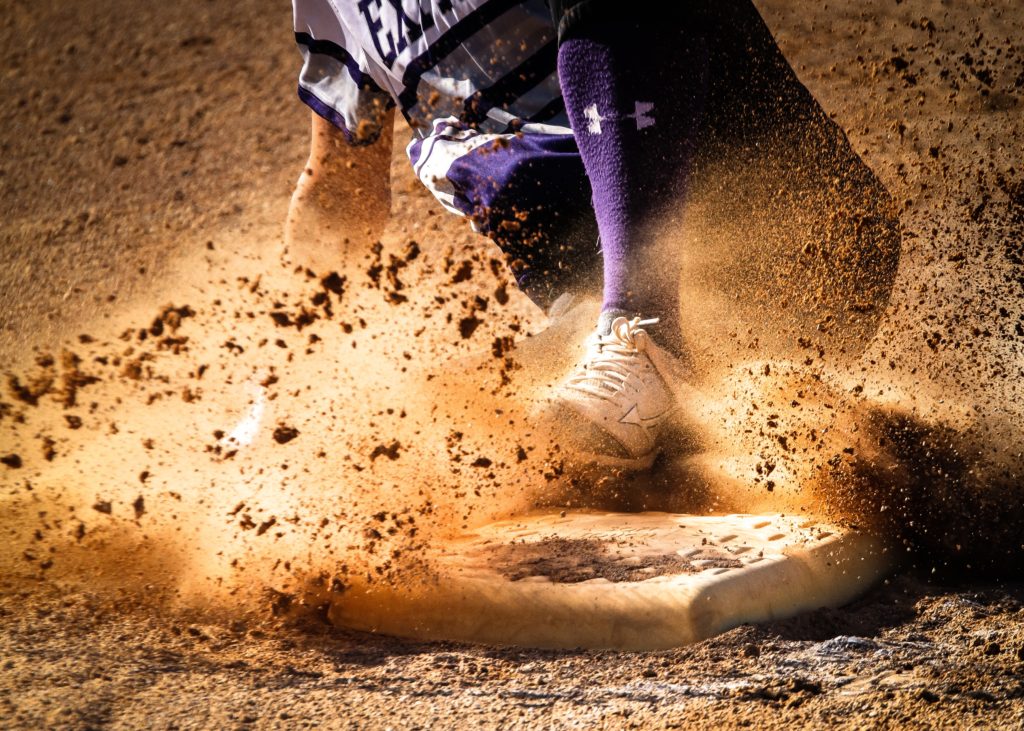
If you want to buy wins, you have to buy runs.
Billy Beane จึงไม่ฟัง คำแนะนำของ แมวมอง และ ได้ทำงานร่วมกับนักวิเคราะห์ข้อมูล Paul Depodesta จากมหาวิทยาลัยฮาวาร์ด
ในหนังใช้ชื่อว่า Peter Brande และจบจากมหาวิทยาลัยเยล แทน เพราะ Paul Depodesta ไม่อยากให้ชื่อตัวเองไปอยู่ในหนัง
Paul วิเคราะห์และพบว่าความสัมพันธ์ระหว่าง ชัยชนะกับ Run ที่ได้ และ Run ที่ได้ต้องได้ Base
ดังนั้นไม่ต้องเลือกผู้เล่นที่ตีลูกแรง, วงสวิงสวย หรือ มีสัดส่วนการตีลูกได้สูง ตามแบบอย่างที่เคยทำมาอย่างที่แมวมองแนะนำ เพราะผู้เล่นเหล่านั้นมีค่าตัวแพง แต่ ต้องการผู้เล่นที่สามารถวิ่งไปที่ Base ได้มากๆ (High On Base Percentage and Slugging Percentage)
และนำมาสู่การตัดสินใจในการเลือกผู้เล่นโดย เลือกผู้เล่นที่ทำ Base ได้สูงสุด และ ราคาค่าตัวถูกสุด
วิธีการทั้งหมดในการวิเคราะห์ข้อมูลผมจะกล่าวถึงรายละเอียดอีกครั้งหนึ่งในหัวข้อ Regression
Billy Beane thinks outside the box. He stops listening to the scout’s suggestions. He starts working with data analyst, Paul DePodesta, from Harvard University.
The concepts of Beane and DePodesta are to use Analytics. To get into Playoffs Oakland A’s must win more than 95 times and get more than 135 Runs is a season.
Paul deeply analyzed and find out that if your need to buy wins, you need to buy runs. If you want to get runs, you have to get base. This means that you don’t need a player who bats strongly, classic swing, or a high percentage of batting but Oakland A’s need the player who has High On Base Percentage and Slugging Percentage.
Paul shares his analysis to Bean to make a decision in choosing players who have high on-base Percentage and Slugging Percentage and Cheapest in the market.
The methodology, Paul using in this analysis, I will get into detail in the topic of Regression.
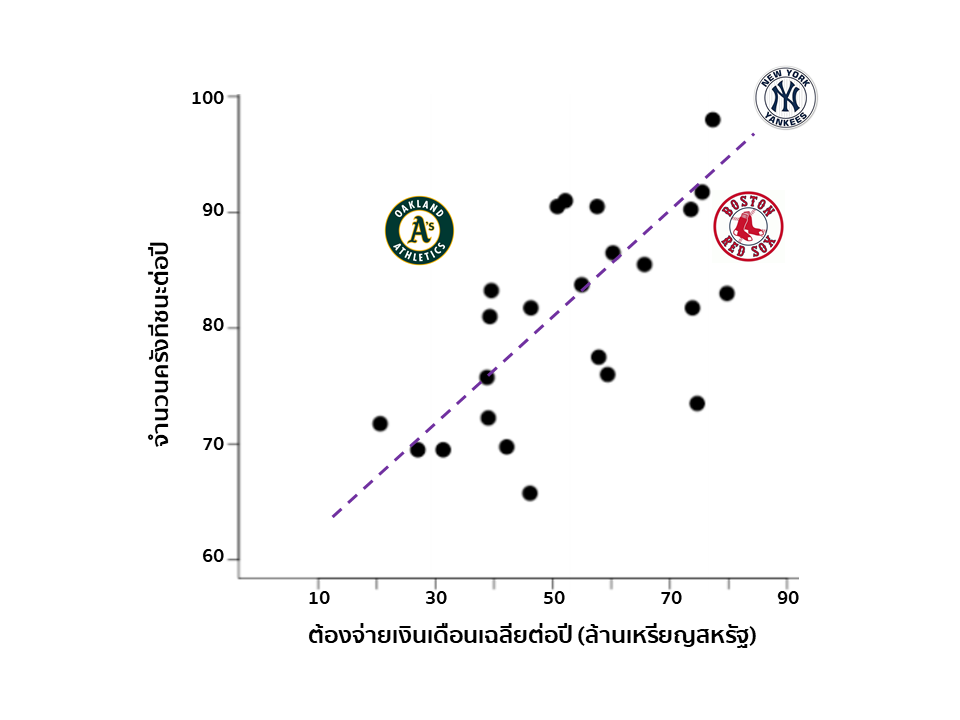
ANALYTICS RESULT
ผลจากการวิเคราะห์ข้อมูลของ Paul แสดงให้เห็นในกราฟว่า Oakland A’s กับ Boston Red Sox สามารถชนะคู่แข่งได้เท่าๆกันคือ ประมาณ 90 ครั้งต่อปี
ในขณะที่ Oakland A’s ใช้จ่ายเงินต่ำกว่า Boston Red Sox ถึง 50 ล้านเหรียญสหรัฐต่อปี ซึ่งเป็นผลมาจาก Analytics
แน่น่อนว่า NewYork Yankee ที่เป็นพ่อบุญทุ่มสามารถชนะได้ถึง 100 matches ต่อปีโดยต้องจ่ายถึง 90 ล้านเหรียญ
และนี่คือบทสรุปที่เห็นได้อย่างชัดเจนในการนำ Analytics มาใช้ในการวิเคราะห์เพื่อการตัดสินใจที่ดีกว่า
Billy Beane and Paul Depodesta have been working together since 1998. The results of using Analytics in Baseball are shown in the above graph. (Data from 1998 – 2001)
The graph shows that Oakland A’s and Boston Red Sox can beat their competitors equally, about 90 times a year.
While Oakland A’s spends less than Boston Red Sox more than $ 50 million a year. This is the result of implementing Analytics in sports
Of course, NewYork Yankee, a rich team, can win up to 100 matches a year, paying $ 90 million.
And this is a clear summary of how Analytics can be used to make better decisions.
อ่านแล้วชอบ เพื่อเป็นกำลังใจ
ช่วย กดLike กดShare ด้วยนะครับ
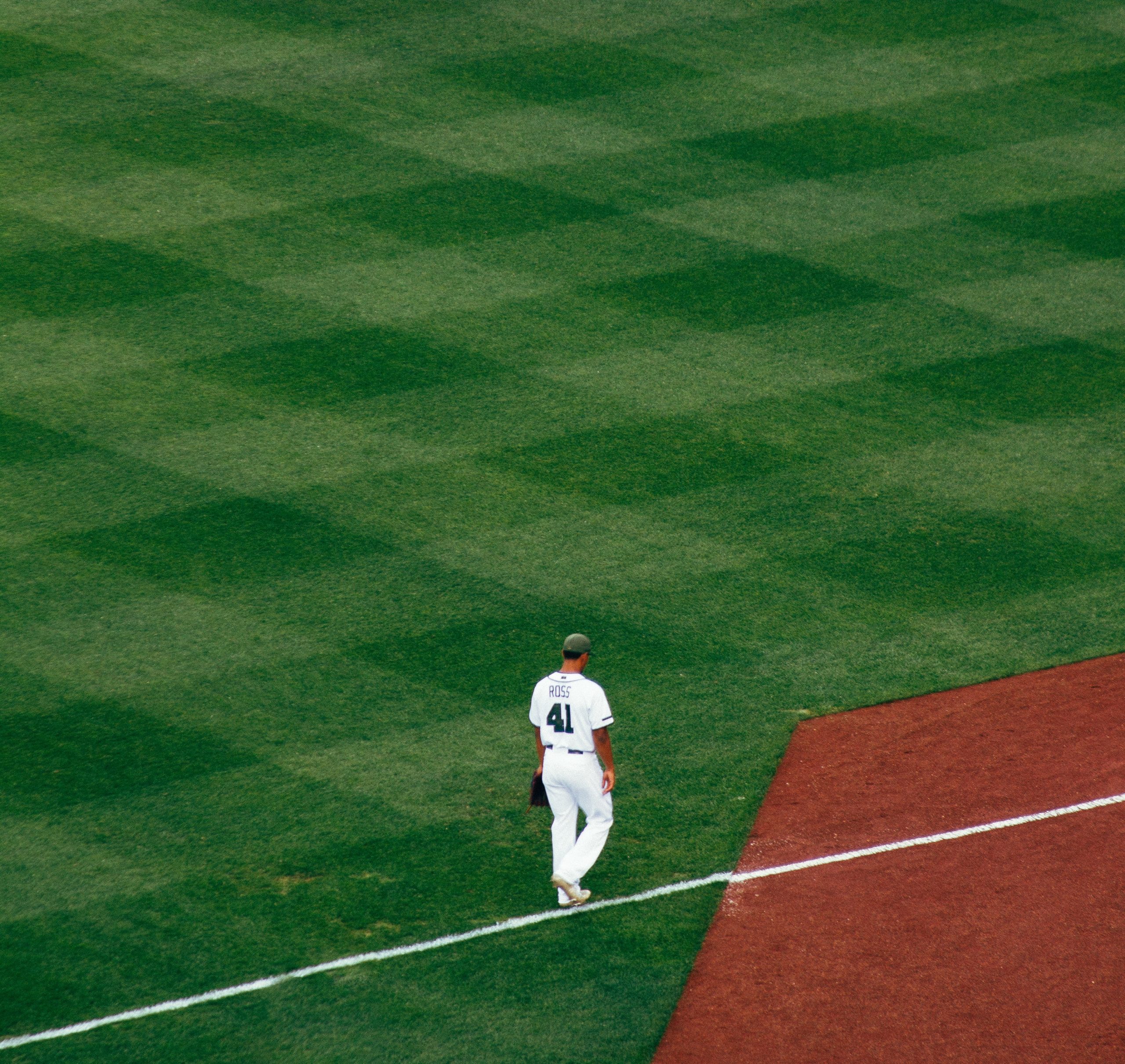
3 Key Players moved to the other teams!
ทีม Oakland A’s ยังคงพบอุปสรรคในการทำทีมในปี 2002 Oakland A’s ต้องเสียผู้เล่นคนสำคัญถึง 3 คนให้ทีมใหญ่ Billy Beane และ Paul Depodesta จะใช้ Analytics แก้ปัญหานี้ได้หรือไม่ อย่างไร ดูในหนังต่อนะครับ
Oakland A’s still faced obstacles. In 2002, Oakland A’s lost 3 key players to big teams. Can Billy Beane and Paul Depodesta use Analytics to solve this problem? How? Please watch the movie.
My Opinion
ผมได้นำเสนอให้เห็นประโยชน์ของการใช้ Analytics ในการกีฬาโดยหยิบยกเหตุการณ์ย้อนหลังไปประมาณเกือบ 20 ปี มาแล้วจากเรื่องราวอันโด่งดังของ หนังสือ Moneyball Art Winning Unfair Game และได้นำมาทำเป็นภาพยนต์ในปี 2011
ปัจจุบันนี้ทุกๆสโมสรจะมีทีมวิเคราะห์ข้อมูลผู้เล่น วิเคราะห์ปัจจัยที่ส่งผลต่อชัยชนะ รวมถึงหลักการและเงื่อนไขในการเลือกผู้เล่นตามข้อมูล
และที่สำคัญ ข้อมูลในปัจจุบันมีความถูกต้องมากขึ้นคือการมีอุปกรณ์ที่ใช้ติดตามตัวผู้เล่น ที่สามารถวัดความเร็วของผู้เล่น อัตราการเต้นของหัวใจ และ ข้อมูลอื่นๆอีกมากมายที่สามารถนำมาวิเคราะห์เพื่อการตัดสินใจในการทำทีม
สุดท้ายนี้ผมอยากแนะนำให้ทุกคนได้ดูหนังเรื่อง Moneyball เพราะหนังเรื่องนี้มีมุมมองมากกว่าการวิเคราะห์ที่ผมนำเสนอแต่มีปรัชญาหลายๆอย่างซ่อนไว้ในหนังที่ทำให้คุณรักภาพยนตร์เรื่องนี้มากขึ้น
I have presented the benefits of using analytics in sports, dating back almost 20 years from the famous Moneyball Art Winning Unfair Game and movies in 2011.
Nowadays, every club has a team to analyze player data. Analyze factors that affect victory Including the principles and conditions for selecting players based on information.
Importantly, the current data is more accurate because of electronic devices to measure the speed, heart rate, steps, and many other data that can be analyzed for team decision making.
Finally, I would like everyone to watch the Moneyball movie because it has more perspectives more than I presented. There are also many philosophies hidden in the movie.